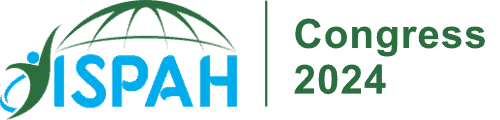
Abstract Overview
Background: Assessing park use is challenging and time-consuming, especially if data is to be gathered over a longer period of time. Digital tools designed to collect long-term data might help us assess activity patterns in less resource-intensive ways.
Purpose: We used an AI-based tool to explore long-term use of a neighborhood activity park.
Methods: In this longitudinal monitoring study, a camera was installed at a new activity park in a Norwegian municipality. The camera has a built-in computer with an embedded AI-solution that quantifies moving objects directly from a video image flow. The AI-solution reads 30 images/second, and moving objects are continuously detected in each image. After detection is completed, images are deleted. Data is automatically transferred to Power BI that manage data using AI-capabilities. Through Power BI, we obtained information on monthly, daily, and hourly passings, and movement tracks. We analyzed data over 98 consecutive days, from 2 October 2023 to 7 January 2024, and linked it to daily weather data from the Norwegian Centre for Climate Services. Descriptive statistics were computed, and heat maps were used to visualize movement patters.
Results: There were 804 daily passings in the park over the period. Highest frequency of activity was observed in the month after opening. In December, we witnessed a substantial drop in daily passing (mean=93), compared to October (mean=1516) and November (mean=1102). There was most activity in the park Fridays to Sundays between 9am-1pm. We observed a change in users’ movement pattern from mid-November, when the weather data indicated a snow depth of 15 cm.
Conclusion: Although a longer observation period is needed, our results indicate that the park is largely used on weekends and that season characteristics influence park use.
Practical implications: An AI-based monitoring tool can be useful for assessing activity in parks.
Funding: Norwegian Research Council.
Additional Authors