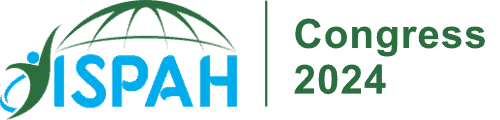
Abstract Overview
Background: The quantity of physical activity one accrues is associated with many health benefits. The quality of physical activity, often operationalised as children’s fundamental movement skill (FMS) proficiency, is also related to health outcomes including physical fitness, weight status, and perceived competence. Traditionally, assessments of FMS are conducted manually by trained raters, which is time consuming and susceptible to biases.
Purpose: To evaluate the performance of an artificial intelligence-based system for FMS assessment.
Methods: FMS performances for jump (n=1,327) and hop (n=1,084) of preschool-aged children (4.51 years, SD=0.79; 57% boys) were video-recorded and scored by a trained rater using the Test of Gross Motor Development version 3 (TGMD-3) criteria. Using these ratings as the ground truth, Skinned Multi-Person Linear models of the performances were extracted and trained using the Long-Short Term Memory architecture, a form of recurring neural network. 80% and 20% of inputted data was used for model training and evaluation, respectively. The percentages of accurate ratings with respect to each TGMD criterion was evaluated.
Results: The overall agreement between model and expert ratings across all tested criteria (four for each skill) ranged between 57% to 93%, with a mean of 75%.
Conclusions: Our results, while only being modest in some criteria, suggest the approach has potential and assessment models for other FMS should be developed and evaluated. Nonetheless, we observed that video resolutions and shooting angles may impact assessment performances. Video-recording protocols would have to be reviewed and modified in order to improve assessment accuracy.
Practical implications: If successful, this system, which is currently linked through a mobile application, could be applied to global research and used as an objective, efficient, and uniform approach for motor skill assessment.
Funding: The project was supported by the Research Impact Fund, University Grants Committee, Hong Kong.
Additional Authors