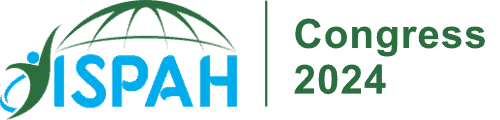
Abstract Overview
Background: Traditionally, existing studies assessing the health associations of accelerometer-measured movement behaviors have been performed with few averaged values, mainly representing the duration of physical activities and sedentary behaviors. Such averaged values cannot naturally capture the complex interplay between the duration, timing, and patterns of accumulation of movement behaviors.
Purpose: To introduce a novel approach to visually represent recorded movement behaviors as images using original accelerometer outputs, allowing the capture of the entire movement behavior profile with information on duration, timing, and patterns in one single image.
Methods: Our method involves converting minute-by-minute accelerometer outputs (activity counts) into a 2D image format, capturing the entire spectrum of movement behaviors performed by each participant. By utilizing convolutional autoencoders, we enable the learning of these image-based representations. Subsequently, we apply the K-means algorithm to cluster these learned representations. We used data from 1812 adult (20-65 years) participants in the National Health and Nutrition Examination Survey (NHANES, 2003-2006 cycles) study who worn a hip-worn accelerometer for 7 seven consecutive days and provided valid accelerometer data.
Results: Deep convolutional autoencoders were able to learn the image representation, encompassing the entire spectrum of movement behaviors. Cluster analysis based on the learned representations for the movement behavior images, resulted in identification of four distinct movement behavior profiles characterized by varying levels, timing, and patterns of accumulation of movement behaviors. These identified profiles were associated with the markers of cardiometabolic health.
Conclusions: Deep learning of movement behavior profiles revealed that, in addition to duration and patterns of movement behaviors, the timing of physical activity may also be crucial for gaining additional health benefits.
Practical implications: The timing of physical activity may also be considered for maximizing the health benefits.
Additional Authors