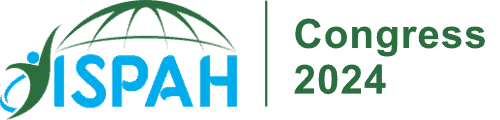
Abstract Overview
Background:
How much time children spent in different postures and movements (PaMs, lying, sitting, standing, walking, running and stair-climbing) is important for their health and development. Thus, accurate measurements of PaMs are essential to understand the daily patterns and their impact on children’s development – information that can be obtained using wearable-based devices, such as accelerometers. While an increasing number of studies are using accelerometry among children, few studies have assessed the validity of machine/deep learning models for identifying a range of PaMs.
Purpose:
The purpose of this study was to evaluate the validity of different machine/deep learning models to recognise PaMs in children between 3 and 14 years old using data from thigh-mounted accelerometers in a laboratory setting.
Methods:
A selection of traditional machine learning models (Unbalanced and Balanced Random Forests, Support Vector Machines, K Nearest Neighbours and XGBoost) and a deep learning model (Long Short-Term Memory-Convolutional Neural Network with an increasing number of layers) were trained using human coded video from 36 children as the reference standard. Models were tested on a further unseen 12 participants.
Results:
Both types of models were found to perform well with the best model for each type having an accuracy ≥ 92% and macro F1 score ≥ 80%. Data processing options such as window length and normalisation method made substantial differences to model performance. Confusion matrices showed that some models performed better with common PaMs (e.g. sitting, lying) and others performed better with less commonly occurring PaMs (e.g. running, stair-climbing).
Conclusions:
Machine learning and deep learning models can accurately classify common postures and movements of children.
Practical implications:
Machine/deep learning can be used for large-scale cohort studies and surveillance to help inform targeted interventions to increase physical activity in children.
Funding:
The Australian Research Council Centre of Excellence for the Digital Child.
Additional Authors